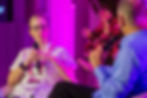
Keynote
NLP-Architect by AI-Lab: An Open Source NLP Library for Developing NLP Solutions
Nowadays, data science teams build a DL model to solve specific NLP tasks. Data-hungry DL models must be fed with large amounts of annotated data, which is time-consuming and costly to acquire since its annotation requires domain expertise. At the same time, business environments are dynamic and complex, making it impractical to collect and label enough data for each scenario in a given domain within a practical time frame. Although much progress has been made in DL for NLP, the current paradigm holdbacks the adoption of NLP based DL/ML in commercial environments. A new set of approaches have emerged with effective transfer learning methods: Embeddings from Language Model (ELMo), Universal Language Model Fine-tuning (ULMFit), Transformer and recently Bidirectional Encoder Representations from Transformers (BERT). These approaches are demonstrating that pre-trained models originally trained on a specific task such as a language model (LM) task can be used successfully for other NLP tasks, outperforming state-of-the-art performance as well as gaining high accuracy with smaller amounts of data when compared to training from scratch In this talk, we present a few solutions and tools based transfer-learning, via NLP Architect library, targeted for a non-DL/NLP expert, that allows to scale and adapt models to new domains by learning from in-domain data with a small amount of labeled examples.
June 26
10 mins
Location
Moshe Wasserblat
Deep Learning and NLP Research Manager, INTEL
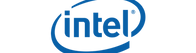